Personalization engines need data to be relevant
Nov 15, 2018 | Website Recommendations, Site Search, E-mail Recommendations
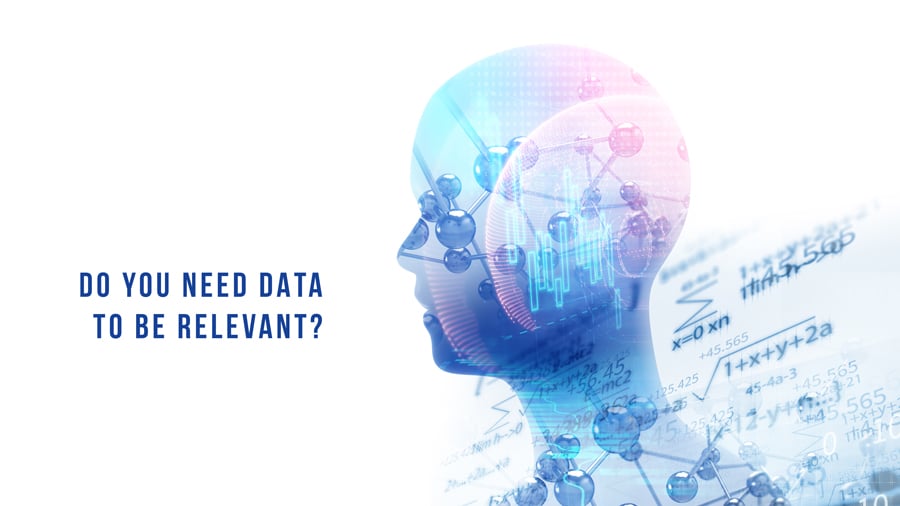
Intelligent data usage is always the foundation of successful personalization solutions.
In the meantime, it is impossible to talk about the intelligent use of data, without also bringing up machine learning, a subset of AI, and a technology that is currently striving towards simulating human thinking.
Machine learning systems automatically improve as the volume of data grows, which is why they are great for e-commerce.
In the e-commerce field, businesses are able to collect enormous amounts of behavioral data and use it to create relevant and personalized user experiences.
Personalization is all about relevance
In creating a relevant online experience, behavioral data is the best starting point. Mining that data enables personalization engines to provide recommendations on anything from content and products, to personalized search results, and even ads.
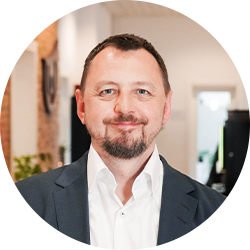
Data is king. You can have the best and most advanced machine learning algorithms running, but without data, it stays dumb. Therefore, every successful implementation starts with evaluating and collecting voluminous and valid data.
Jan Skov
COO, Raptor Services
Algorithms that learn from data will also improve their output as they process more data and form patterns. This performance case on Bog&idé perfectly reflects this statement.
Read more: Case Study – Bog & idé: More Inside
Tuning an algorithm from a human perspective
The condition for a child to be able to distinguish a cat from a dog is that the child sees examples of both animals and recognizes their differences.
Just as a newborn human requires various inputs to develop and learn, an algorithm needs input to identify meaningful patterns in data.
Personalization engines create these patterns from user behavior on a website or another channel. When relations are established between items and user behavior, such as purchases, the algorithm is able to provide users with recommendations that assist them with both explicit and latent needs.
For personalization to perform at the highest level, large amounts of data are vital. The more data, the more precise the recommendations!
Overcoming the challenge of sparse data
At Raptor we use various data-ingests, eg. a real-time data stream of behavioral data. To be precise, we stream data from several digital touchpoints such as web, email, social media, banner, and display.
However, some items or pieces of content have very little online user data attached to them. An example could be a product on a webshop that is not sold or viewed very frequently.
Read more: Recommend products in your content to increase sales
For a recommendation engine, sparse data means that it will be unable to recognize relevant patterns from behavioral data on such long-tail items.
Therefore, personalization providers have to develop strategies to overcome sparse data issues:
- For example, algorithms can be set to detect user behavior from a product’s taxonomy, rather than from the product itself. In this case, the algorithm would recommend items or pieces of content that are relevant in relation to a category rather than a specific product.
- It is also possible to configure an algorithm to learn from semantics, rather than user behavior. In that case, recommendations would be made on the basis of keywords, headlines, and descriptions.
- Finally, some retailers have goldmines of transactional data from physical stores, which can be used to enrich online recommendations and increase the relevance towards the user.
- Personalization engines need data to form meaningful patterns and calculate relevant recommendations.
Related Content
You might also like
Is this how you create newsletters too? If you work in e-commerce, you might recognize this routine:
Learn More
No reason to sugarcoat it. E-commerce is facing a lot of challenges right now. Chinese giants like...
Learn More
It’s a name that’s impossible to ignore. No matter how hard you try. TEMU. The Chinese giant has...
Learn More
Are you looking to take your e-mail marketing to the next level and increase conversion rates? Then...
Learn More
It’s a common myth that B2B Sales don’t really need a proper e-commerce setup. After all, “people...
Learn More
In the dynamic world of digital interactions, businesses are constantly striving to capture the...
Learn More
In today’s E-commerce landscape, Website Personalization is completely essential to drive...
Learn More
Do you transform your Black Friday encounters into lasting relationships? Or do you let this annual...
Learn More
What is Site Search in E-commerce? Site search in E-commerce is when a search engine is integrated...
Learn More
Raptor is proud to present our most advanced recommendation module to date. Find out what the new...
Learn More


Let us show you what you can achieve with premium personalization
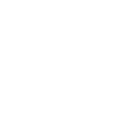
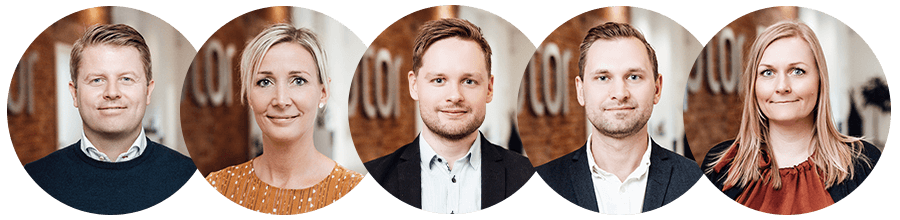
A Raptor expert can share more about the product and answer any questions you have.